10 Lessons for Smarter AI Adoption – A Roadmap to Success
- Manoj Mathen
- Dec 31, 2024
- 3 min read
Updated: Jan 17
AI and Data Science are evolving faster than ever, offering immense potential for transformation. Yet, after working closely with several organizations in 2024, I’ve seen how at times, even the most promising projects can falter—not because the tech isn’t ready, but due to execution gaps. Here are my 10 hard-earned lessons and actionable fixes to help you turn AI challenges into success stories. #AIAdoption #AI #machinelearning #enterpriseAI
Which of these challenges resonates most with your experience? Have you faced any others I haven’t listed? Let’s discuss below!
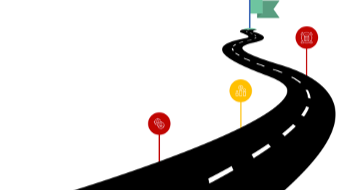
December 31, 2024
AI and Data Science are evolving faster than ever, offering immense potential for transformation. Yet, after working closely with several organizations in 2024, I’ve seen how at times, even the most promising projects can falter—not because the tech isn’t ready, but due to execution gaps. Here are few of my 10 hard-earned lessons and actionable fixes to help you turn AI challenges into success stories.
🚫Lack of Clear Business Goals – Cool tech without clear objectives equals zero ROI. ✅ Tip: Start with the problem/objective, not the tool. Tie every AI project to measurable KPIs upfront.
📊 Data Quality Issues – AI is only as good as the data it learns from. Incomplete, biased, or messy data spells disaster. ✅ Tip: Invest in data profiling and cleansing processes early. Real world data is messy. Use automated tools to handle missing values and outliers.
🧠 Overlooking Domain Expertise – Models require more than algorithms- they need the domain flavours. Without them, AI might detect patterns but miss context. ✅ Tip: Collaborate closely with domain experts during model design to capture business context and refine features.
🤹 Model Overfitting – Models that look great in testing often fail in the real world because they learn too much noise. ✅ Tip: Always test on unseen data. Use techniques like cross-validation, regularization, and dropouts to prevent overfitting.
💸 Underestimating Costs – Hidden costs in data preparation, infrastructure, and model retraining can sink projects. Budgeting only for development is a costly mistake. ✅ Tip: Plan for end-to-end costs, including infrastructure, retraining, and post-deployment monitoring in the budget.
👥 Talent Shortage – Lack of skilled AI engineers, MLOps specialists, and data scientists often stalls progress. ✅ Tip: Build cross-functional teams with data engineers, MLOps specialists, and business analysts for holistic execution.
📉 Poor Change Management – People fear what they don’t understand. Adoption fails without proper training and buy-in. ✅ Tip: Educate stakeholders early, communicate wins clearly, and provide hands-on training to drive adoption.
⏳ Slow Deployment Cycles – AI models that stay in labs never make it to production. Speed matters! ✅ Tip: Use MLOPs , CI/CD pipelines to automate testing, deployment, and scaling of AI models.
📡 Ignoring Monitoring & Maintenance – AI isn’t “set it and forget it.” Models degrade over time and need ongoing tuning. ✅ Tip: Implement automated monitoring for model performance, data/model drift, and fairness metrics to ensure long-term stability.
⚖️ Ethical Concerns – Privacy breaches, algorithmic bias, and fairness concerns can damage reputation and trust. ✅ Tip: Conduct fairness audits, bias testing, and ensure transparency in decision-making to build trust and compliance.
💼 Bottom Line: AI isn’t magic—it’s a journey. Success demands strategy, execution, and alignment with business priorities. With the right approach, these challenges can become stepping stones for impactful AI adoption
Which of these challenges resonates most with your experience? Have you faced any others I haven’t listed? Let’s discuss below!
Comentarios